- Phil Bianco, Chief Technology Officer,
- linkedin.com/in/philbianco/
- July 26, 2023
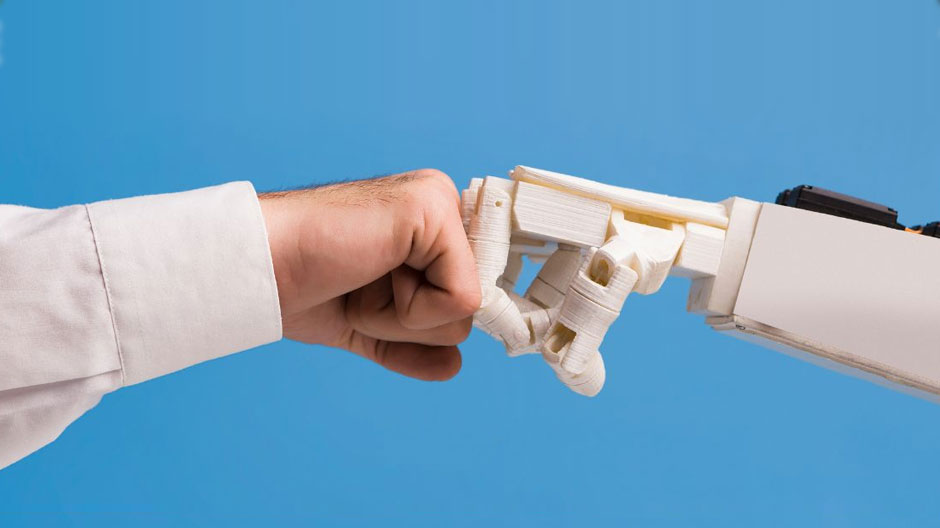
ChatGPT and other large language model artificial intelligence (LLM AI) models are getting a lot of attention for their ability to develop content and images. Now business across multiple industries want to incorporate LLM AIs into their daily workflows. That’s possible today, because the level of computing power that used to be accessible only by government agencies and research scientists is now available to every business.
For example, HPE recently introduced HPE GreenLake for Large Language Models (LLMs) enabling businesses to train, tune and deploy large-scale AI through its GreenLake cloud service. And these new capabilities will also be available as industry-specific applications in the future. HPE has said it plans to roll out applications for supporting climate modeling, healthcare and life sciences, financial services, manufacturing, and transportation. By incorporating new AI capabilities into its GreenLake environment and toolsets, HPE is building the foundation for fueling future innovation.
Consider what this can mean for your business. It is now financially cost effective to use AI to define and build solutions, based on your existing data, for existing problems as well as creating new revenue streams. Before you can build an AI model for your data, you need to take a few steps to determine exactly what you want to achieve.
- Clearly define your specific business objective. This could be creating a new AI solution to become a force multiplier for your business. It could also be trend analysis and heat mapping associated with business expansion into underpenetrated markets. Whatever the business problem, it must be clearly defined and scoped.
- Define the appropriate data sets related to your business challenge. AI is great at correlating data from disparate systems. You can compare payment history from your customer base with traffic flows and environmental factors to determine the best new markets for opening stores. Whatever the specific case, you need to determine the most useful and appropriate data sets you have available.
- Determine data congruency across your data sets. By data congruency, I mean ensuring that your data sets are mapped correctly. For example, in one data set you may have Field A equal to First Name while in another data set, Field A is equal to Company Name. Another area to review is data precision, where one data set might be using percent symbols while another uses decimal to three places. Your data sets must be congruent and aligned before you can use it for analysis. This might also be a small area where you can apply AI to achieve a specific goal of cleaning and aligning your data before it is fed into your data lake for your larger solution.
Once you’ve taken these steps, you can build an AI model to achieve your business goals or solve your business challenges.
Decreasing Time and Increasing Accuracy through LLM AI
One of our financial institution clients prepares Suspicious Activity Reports (SARs) for filing with the Financial Crimes Enforcement Network (FinCEN), the regulatory body for this instance. Using the steps above, the client was able to define a specific business objective, define the data sets required for success and determine data congruency for disparate data sets.
What used to take over an hour to write now takes about 30 seconds to create by leveraging a large language model (LLM) AI tool. If potentially fraudulent activity is flagged, a case worker investigates and completes a form containing a set of standard questions used to form a data set. This generates a two-page SAR for regulators. If there is an issue, the investigator can quickly update the document. Once he’s satisfied with the report, he can send it right through to the regulator. In addition to being sent to the regulator, the data is fed back into the LLM to help improve the model for other inquiries.
The steps are largely the same, but the use of the AI tool reduces errors, speeds along the process, and helps improve the process as well.
In the case of GreenLake, HPE is using an LLM from Aleph Alpha which enables you to leverage your organization’s data and then train and fine-tune a customized AI model to obtain real-time insights based on your own proprietary knowledge. You can then integrate AI applications you build into your workflows.
From a business perspective, this makes sense – you are now able to better utilize data sets that you collect from across the organization to develop innovative solutions for business challenges and growth. From an IT perspective, this also makes sense as well. You can still support the traditional technology workloads for your business, including storage and compute. And within the GreenLake framework you can also integrate AI workloads. Now you have one management layer across all those disparate workloads.